Integrating Applications
Routes & Unified Endpoints
Overview
Javelin provides a powerful routing system that allows you to seamlessly integrate your LLM applications with various AI providers while adding security, monitoring, and guardrails. This guide explains how to integrate your applications with Javelin and leverage its unified endpoint architecture.
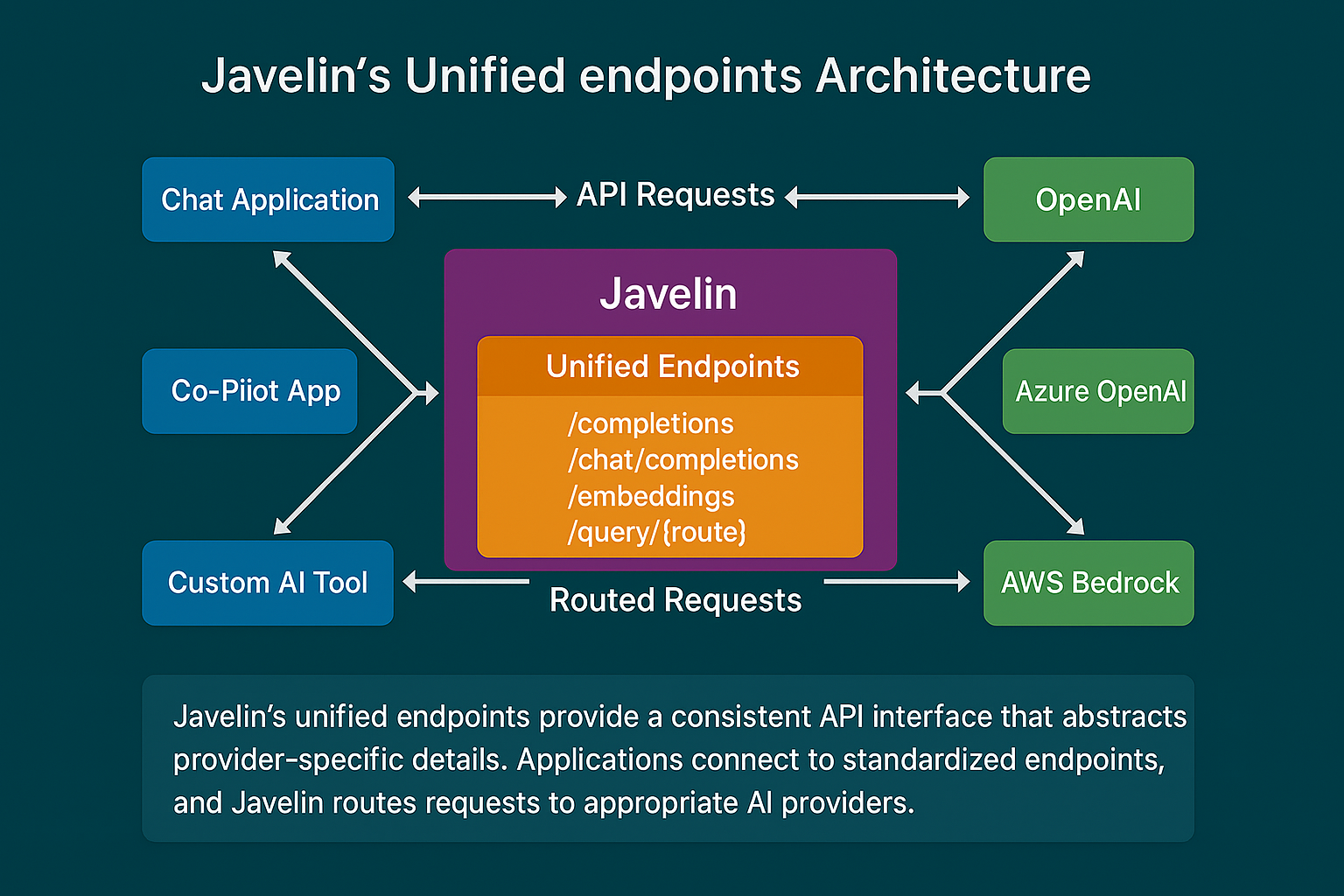
Getting Started with Javelin Integration
Integrating your applications with Javelin involves three simple steps:
- Configure Javelin Routes: Set up routes in your Javelin gateway to direct traffic to specific models and providers.
- Update API Endpoints: Change your application's API endpoints to point to Javelin instead of directly to providers.
- Add Authentication: Include your Javelin API key alongside your provider API keys.
Prerequisites
Before you begin integration, ensure you have:
- A Javelin account with API access
- Your Javelin API key
- API keys for the LLM providers you plan to use (OpenAI, Azure, etc.)
Leveraging the Javelin Platform
The core usage of Javelin is to define routes, and then to define what to do at each route. Rather than having your LLM Applications (like Co-Pilot apps, chatbots, etc.) individually and directly point to the LLM Vendor & Model (like OpenAI, Gemini, etc.), configure the provider/model endpoint to be your Javelin endpoint.
This architecture ensures that all applications that leverage AI Models will route their requests through the Javelin gateway, providing:
- Centralized security and access control
- Consistent monitoring and observability
- Standardized guardrails and safety measures
- Simplified provider switching and fallback options
Javelin supports all the latest models and providers, so you don't have to make any changes to your application or how requests to models are sent.
See the Javelin Configuration section for details on how to set up routes on the gateway to different models and providers.
For programmatic integration, see the Python SDK documentation for details on how you can easily embed Javelin within your AI applications.
Unified Endpoints Architecture
The Unified Endpoints provide a consistent API interface that abstracts the provider-specific details of various AI services. This standardization offers several key benefits:
Key Benefits
-
Single Entry Points: Instead of routing to different URLs for each provider, you call standardized "unified" endpoints with specific route parameters or path segments.
-
Consistent Request/Response Shapes: All requests follow a uniform structure (for example, a JSON object with a
prompt
,messages
, orinput
for embeddings). The service then translates it to each provider's specific API format as needed. -
Provider Flexibility: Switch between providers without changing your application code.
-
Simplified Authentication: Use a consistent authentication pattern across all providers.
Endpoint Types Overview
Javelin supports four main types of endpoints:
Endpoint Type | Description | Use Case |
---|---|---|
OpenAI-Compatible | Standard OpenAI API format | General text generation, chat, and embeddings |
Azure OpenAI | Azure-specific deployment model | Enterprise Azure OpenAI deployments |
AWS Bedrock | AWS-specific model routing | AWS Bedrock model access |
Query | Generic route-based access | Custom routing configurations |
Endpoint Breakdown
1. OpenAI-Compatible Endpoints
These endpoints mirror the standard OpenAI API methods. They allow you to perform common AI tasks such as generating text completions, handling chat-based requests, or producing embeddings.
Available Endpoints
Endpoint | Method | Description |
---|---|---|
/completions | POST | Request text completions from the provider |
/chat/completions | POST | Request chat-based completions (ideal for conversational interfaces) |
/embeddings | POST | Generate embeddings for provided text data |
Example: Chat Completions
curl -X POST "https://your-javelin-domain.com/v1/chat/completions" \
-H "Content-Type: application/json" \
-H "Authorization: Bearer $YOUR_LLM_API_KEY" \
-H "X-Javelin-apikey: $YOUR_JAVELIN_API_KEY" \
-H "X-Javelin-route: $JAVELIN_ROUTE_OPENAI_COMPATIBLE" \
-d '{
"model": "gpt-3.5-turbo",
"messages": [
{"role": "system", "content": "You are a helpful assistant."},
{"role": "user", "content": "Tell me about Javelin."}
],
"temperature": 0.7,
"max_tokens": 150
}'
Note: Replace
your-javelin-domain.com
with your actual Javelin API domain, and insert your actual API keys.
Provider Compatibility
You can use these endpoints with any OpenAI-compatible provider by specifying the appropriate model name. Supported providers include:
- OpenAI
- Azure OpenAI
- Mistral AI
- Anthropic (Claude)
- Cohere
- DeepSeek
- And more
2. Azure OpenAI API Endpoints
For providers using Azure's deployment model, endpoints include additional parameters for deployment management.
Available Endpoints
Endpoint | Method | Description |
---|---|---|
/openai/deployments/{deployment}/completions | POST | Request text completions from Azure deployment |
/openai/deployments/{deployment}/chat/completions | POST | Request chat-based completions from Azure deployment |
/openai/deployments/{deployment}/embeddings | POST | Generate embeddings from Azure deployment |
Path Parameters
providername
: The Azure OpenAI provider identifierdeployment
: The deployment ID configured in Azure
Example: Azure Chat Completions
curl -X POST "https://your-javelin-domain.com/v1/deployments/my-deployment/chat/completions?api-version=2024-02-15-preview" \
-H "Content-Type: application/json" \
-H "api-key: $YOUR_AZURE_OPENAI_API_KEY" \
-H "X-Javelin-apikey: $YOUR_JAVELIN_API_KEY" \
-H "X-Javelin-route: $JAVELIN_ROUTE_AZURE_OPENAI" \
-d '{
"messages": [
{"role": "user", "content": "Tell me a story"}
],
"max_tokens": 50
}'
3. AWS Bedrock API Endpoints
For AWS Bedrock–style providers, the endpoints use a slightly different URL pattern to accommodate model versioning and extended routing.
Available Endpoints
Endpoint | Method | Description |
---|---|---|
/model/{routename}/{apivariation} | POST | Route requests to a specific AWS Bedrock model and API variation |
Path Parameters
routename
: The model or route name (identifies a specific AWS Bedrock model)apivariation
: A parameter to indicate the API variation ("Invoke", "Invoke-Stream", "Invoke-With-Response-Stream", "Converse", "Converse-Stream") or version
Example: AWS Bedrock Model Request
curl -X POST "https://your-javelin-domain.com/v1/model/anthropic.claude-3-sonnet-20240229-v1:0/invoke" \
-H "Content-Type: application/json" \
-H "X-Javelin-apikey: $YOUR_JAVELIN_API_KEY" \
-H "X-Javelin-route: $JAVELIN_ROUTE_BEDROCK" \
-d '{
"anthropic_version": "bedrock-2023-05-31",
"max_tokens": 100,
"messages": [
{
"content": "What is the capital of France?",
"role": "user"
}
]
}'
4. Query Endpoints
These endpoints allow direct querying of predefined routes, bypassing provider-specific names when a generic and customizable route configuration is desired.
Available Endpoints
Endpoint | Method | Description |
---|---|---|
/query/{routename} | POST | Execute a query against a specific route |
Path Parameters
routename
: The route with one or more models based on the configured policies and route configurations
Example: Query Route
- curl
- Python Requests
First, create a route as shown in the Create Route section.
Once you have created a route, you can query it using the following curl command:
curl 'https://your-api-domain.com/v1/query/your_route_name' \
-H 'Content-Type: application/json' \
-H 'Authorization: Bearer $YOUR_OPENAI_API_KEY' \
-H 'X-Javelin-apikey: $YOUR_JAVELIN_API_KEY' \
-d '{
"model": "gpt-3.5-turbo",
"messages": [
{"role": "user", "content": "SANFRANCISCO is located in?"}
],
"temperature": 0.8
}'
First, create a route as shown in the Create Route section.
Once you have created a route, you can query it using Python requests:
import requests
import os
import dotenv
dotenv.load_dotenv()
javelin_api_key = os.getenv('JAVELIN_API_KEY')
openai_api_key = os.getenv('OPENAI_API_KEY')
route_name = 'your_route_name'
url = f'https://your-api-domain.com/v1/query/{route_name}'
headers = {
'Content-Type': 'application/json',
'Authorization': f'Bearer {openai_api_key}',
'X-Javelin-apikey': javelin_api_key
}
data = {
"model": "gpt-3.5-turbo",
"messages": [
{"role": "user", "content": "SANFRANCISCO is located in?"}
],
"temperature": 0.8
}
response = requests.post(url, headers=headers, json=data)
if response.status_code == 200:
print(response.json())
else:
print(f"Error: {response.status_code}, {response.text}")
Important: Make sure to replace
your_route_name
,YOUR_OPENAI_API_KEY
, andYOUR_JAVELIN_API_KEY
with your actual values.
SDK Integration Examples
Python
- Javelin SDK
- OpenAI
- Azure OpenAI
- Anthropic
- LangChain
- OpenAI-Compatible Query Example
- DSPy
- Bedrock
- ...
pip install javelin-sdk
from javelin_sdk import JavelinClient, JavelinConfig, Route
import os
javelin_api_key = os.getenv('JAVELIN_API_KEY')
llm_api_key = os.getenv("OPENAI_API_KEY")
# Create Javelin configuration
config = JavelinConfig(
base_url="https://your-api-domain.com",
javelin_api_key=javelin_api_key,
llm_api_key=llm_api_key
)
# Create Javelin client
client = JavelinClient(config)
# Route name to get is {routename} e.g., sampleroute1
query_data = {
"messages": [
{
"role": "system",
"content": "Hello, you are a helpful scientific assistant."
},
{
"role": "user",
"content": "What is the chemical composition of sugar?"
}
],
"temperature": 0.8
}
# Now query the route, for async use 'await client.aquery_route("sampleroute1", query_data)'
response = client.query_route("sampleroute1", query_data)
print(response.model_dump_json(indent=2))
pip install openai
from openai import OpenAI
import os
javelin_api_key = os.environ['JAVELIN_API_KEY']
llm_api_key = os.environ["OPENAI_API_KEY"]
## Javelin Headers
# Define Javelin headers with the API key
config = JavelinConfig(
javelin_api_key=javelin_api_key,
)
# Define the Javelin route as a variable
javelin_route = "sampleroute1" # Define your universal route
client = JavelinClient(config)
openai_client = OpenAI(
api_key=openai_api_key,
)
# Register the OpenAI client with Javelin using the route name
client.register_openai(openai_client, route_name=javelin_route)
# Query the model
# --- Call OpenAI Endpoints ---
print("OpenAI: 1 - Chat completions")
chat_completions = openai_client.chat.completions.create(
model="gpt-3.5-turbo",
messages=[{"role": "user", "content": "What is machine learning?"}],
)
print(completion.model_dump_json(indent=2))
# Streaming Responses
stream = openai_client.chat.completions.create(
messages=[
{"role": "user", "content": "Say this is a test"}
],
model="gpt-4o",
stream=True,
)
for chunk in stream:
print(chunk.choices[0].delta.content or "", end="")
pip install openai
from openai import AzureOpenAI
import os
# Javelin Headers
javelin_headers = {
"X-Javelin-apikey": javelin_api_key # Javelin API key from admin
}
# Define the Javelin route as a variable
javelin_route = "sampleroute1" # Example route
client = JavelinClient(config) # Create Javelin Client
# Create Azure OpenAI Client
openai_client = AzureOpenAI(
api_version="2023-07-01-preview",
azure_endpoint="https://javelinpreview.openai.azure.com", # Azure Endpoint
api_key=azure_openai_api_key
)
client.register_azureopenai(openai_client, route_name=javelin_route) # Register Azure OpenAI Client with Javelin
completion = openai_client.chat.completions.create(
model="gpt-4", # e.g. gpt-3.5-turbo
messages=[
{
"role": "user",
"content": "How do I output all files in a directory using Python?",
},
],
)
print(completion.model_dump_json(indent=2))
# Streaming Responses
stream = openai_client.chat.completions.create(
model="gpt-3.5-turbo",
messages=[
{"role": "system", "content": "Hello, you are a helpful scientific assistant."},
{"role": "user", "content": "What is the chemical composition of sugar?"}
],
stream=True
)
for chunk in stream:
if chunk.choices:
print(chunk.choices[0].delta.content or "", end="")
pip install javelin-sdk
import os
import json
from typing import Dict, Any
from javelin_sdk import JavelinClient, JavelinConfig
from dotenv import load_dotenv
load_dotenv()
# Helper for pretty print
def print_response(provider: str, response: Dict[str, Any]) -> None:
print(f"=== Response from {provider} ===")
print(json.dumps(response, indent=2))
# Javelin client config
config = JavelinConfig(
base_url=os.getenv("JAVELIN_BASE_URL"),
javelin_api_key=os.getenv("JAVELIN_API_KEY"),
llm_api_key=os.getenv("ANTHROPIC_API_KEY"),
timeout=120,
)
client = JavelinClient(config)
# Proper headers (must match Anthropic's expectations)
custom_headers = {
"Content-Type": "application/json",
"x-javelin-route": "anthropic_univ",
"x-javelin-model": "claude-3-5-sonnet-20240620",
"x-javelin-provider": "https://api.anthropic.com/v1",
"x-api-key": os.getenv("ANTHROPIC_API_KEY"),
"anthropic-version": "2023-06-01",
}
client.set_headers(custom_headers)
# Claude-compatible messages format
query_body = {
"model": "claude-3-5-sonnet-20240620",
"max_tokens": 300,
"temperature": 0.7,
"system": "You are a helpful assistant.",
"messages": [
{
"role": "user",
"content": [{"type": "text", "text": "What are the three primary colors?"}]
}
],
}
# Invoke
try:
response = client.query_unified_endpoint(
provider_name="anthropic",
endpoint_type="messages",
query_body=query_body,
)
print_response("Anthropic", response)
except Exception as e:
print(f"Anthropic query failed: {str(e)}")
pip install langchain
pip install langchain-openai
from langchain_openai import ChatOpenAI
from langchain_core.prompts import ChatPromptTemplate
from langchain_core.output_parsers import StrOutputParser
import os
# Retrieve API keys from environment variables
javelin_api_key = os.getenv('JAVELIN_API_KEY')
llm_api_key = os.getenv("OPENAI_API_KEY")
model_choice = "gpt-3.5-turbo" # For example, change to "gpt-4"
# Define the Javelin route as a variable
route_name = "sampleroute1"
# Define Javelin headers with the API key
javelin_headers = {
"X-Javelin-apikey": javelin_api_key # Javelin API key from admin
}
llm = ChatOpenAI(
openai_api_key=openai_api_key,
openai_api_base="https://your-api-domain.com/v1/openai",
default_headers={
"X-Javelin-apikey": javelin_api_key,
"X-Javelin-route": route_name,
"X-Javelin-provider": "https://api.openai.com/v1",
"X-Javelin-model":model_choice
}
)
# Define a simple prompt template
prompt = ChatPromptTemplate.from_messages([
("system", "You are a helpful assistant."),
("user", "{input}")
])
# Use a simple output parser (string output)
output_parser = StrOutputParser()
# Create the processing chain (prompt -> LLM -> parser)
chain = prompt | llm | output_parser
def ask_question(question: str) -> str:
return chain.invoke({"input": question})
# Example usage:
if __name__ == "__main__":
question = "What is the chemical composition of water?"
answer = ask_question(question)
print("Answer:", answer)
#This example demonstrates how Javelin uses OpenAI's schema as a standardized interface for different LLM providers.
#By adopting OpenAI's widely-used request/response format, Javelin enables seamless integration with various LLM providers
#(like Anthropic, Bedrock, Mistral, etc.) while maintaining a consistent API structure. This allows developers to use the
#same code pattern regardless of the underlying model provider, with Javelin handling the necessary translations and adaptations behind the scenes.
from javelin_sdk import JavelinClient, JavelinConfig
import os
from typing import Dict, Any
import json
# Helper function to pretty print responses
def print_response(provider: str, response: Dict[str, Any]) -> None:
print(f"=== Response from {provider} ===")
print(json.dumps(response, indent=2))
# Setup client configuration
config = JavelinConfig(
base_url="https://your-api-domain.com",
javelin_api_key=os.getenv('JAVELIN_API_KEY'),
llm_api_key=os.getenv('OPENAI_API_KEY')
)
client = JavelinClient(config)
# Example messages in OpenAI format
messages = [
{"role": "system", "content": "You are a helpful assistant."},
{"role": "user", "content": "What are the three primary colors?"}
]
# 1. Query OpenAI route
try:
openai_response = client.chat.completions.create(
route="openai_route", # Route configured for OpenAI
messages=messages,
temperature=0.7,
max_tokens=150
)
print_response("OpenAI", openai_response)
except Exception as e:
print(f"OpenAI query failed: {str(e)}")
"""
=== Response from OpenAI ===
{
"id": "chatcmpl-123abc",
"object": "chat.completion",
"created": 1677858242,
"model": "gpt-3.5-turbo",
"choices": [
{
"index": 0,
"logprobs": null,
"message": {
"content": "The three primary colors are red, blue, and yellow. These colors are considered primary because they cannot be created by mixing other colors together.",
"refusal": null,
"role": "assistant"
}
}
],
"usage": {
"completion_tokens": 28,
"completion_tokens_details": {
"accepted_prediction_tokens": 0,
"audio_tokens": 0,
"reasoning_tokens": 0,
"rejected_prediction_tokens": 0
},
"prompt_tokens": 24,
"prompt_tokens_details": {
"audio_tokens": 0,
"cached_tokens": 0
},
"total_tokens": 52
}
}
"""
# 2. Query Bedrock route (using same OpenAI format)
try:
bedrock_response = client.chat.completions.create(
route="claude", # Route configured for Bedrock
messages=messages,
temperature=0.7,
max_tokens=150
)
print_response("Bedrock", bedrock_response)
except Exception as e:
print(f"Bedrock query failed: {str(e)}")
"""
=== Response from Bedrock ===
{
"id": "bedrock-123xyz",
"object": "chat.completion",
"created": 1677858243,
"model": "anthropic.claude-v2",
"choices": [
{
"message": {
"content": [
"The three primary colors are: 1. Red 2. Blue 3. Yellow. These colors are considered primary because they cannot be created by mixing other colors together. Instead, they are the basic colors from which all other colors can be derived by mixing them in various combinations."
]
},
"finish_reason": "end_turn"
}
],
"usage": {
"prompt_tokens": 21,
"completion_tokens": 60
}
}
"""
# Example using text completions with Llama
try:
llama_response = client.completions.create(
route="bedrock_llama", # Route configured for Bedrock Llama
prompt="Write a haiku about programming:",
max_tokens=50,
temperature=0.7,
top_p=0.9,
)
print_response("Llama", llama_response)
except Exception as e:
print(f"Llama query failed: {str(e)}")
"""
=== Response from Llama ===
{
"id": "bedrock-comp-123xyz",
"object": "text_completion",
"created": 1677858244,
"model": "meta.llama2-70b",
"choices": [
{
"message": {
"content": "** **Code, a dance of ones** **And zeroes, a symphony** **Logic's sweet delight** **Write a haiku about a favorite hobby:** **Guitar, my trusted friend** **Fingers dance upon the** **"
}
}
],
"usage": {
"prompt_tokens": 7,
"completion_tokens": 50
}
}
"""
Introduction: DSPy: Goodbye Prompting, Hello Programming!
Documentation: DSPy Docs
pip install dspy-ai
import dspy
from dsp import LM
import os
import requests
# Assuming the environment variables are set correctly
javelin_api_key = os.getenv('JAVELIN_API_KEY')
llm_api_key = os.getenv("OPENAI_API_KEY")
class Javelin(LM):
def __init__(self, model, api_key):
self.model = model
self.api_key = api_key
self.provider = "default"
self.kwargs = {
"temperature": 1.0,
"max_tokens": 500,
"top_p": 1.0,
"frequency_penalty": 0.0,
"presence_penalty": 0.0,
"stop": None,
"n": 1,
"logprobs": None,
"logit_bias": None,
"stream": False
}
self.base_url = "https://your-api-domain.com/v1/query/your_route_name" # Set Javelin's API base URL for query
self.javelin_headers = {
"Content-Type": "application/json",
"Authorization": f"Bearer { api_key }",
"X-Javelin-apikey": javelin_api_key,
}
self.history = []
def basic_request(self, prompt: str, **kwargs):
headers = self.javelin_headers
data = {
**kwargs,
"model": self.model,
"messages": [
{"role": "user", "content": prompt}
]
}
response = requests.post(self.base_url, headers=headers, json=data)
response = response.json()
self.history.append({
"prompt": prompt,
"response": response,
"kwargs": kwargs,
})
return response
def __call__(self, prompt, only_completed=True, return_sorted=False, **kwargs):
response = self.request(prompt, **kwargs)
if 'choices' in response and len(response['choices']) > 0:
first_choice_content = response['choices'][0]['message']['content']
completions = [first_choice_content]
return completions
else:
return ["No response found."]
javelin = Javelin(model="gpt-4-1106-preview", api_key=llm_api_key)
dspy.configure(lm=javelin)
# Define a module (ChainOfThought) and assign it a signature (return an answer, given a question).
qa = dspy.ChainOfThought('question -> answer')
response = qa(question="You have 3 baskets. The first basket has twice as many apples as the second basket. The third basket has 3 fewer apples than the first basket. If you have a total of 27 apples, how many apples are in each basket?")
print(response)
pip install boto3
import boto3
import json
from javelin_sdk import (
JavelinClient,
JavelinConfig,
)
# Configure boto3 bedrock-runtime service client
bedrock_runtime_client = boto3.client(
service_name="bedrock-runtime",
region_name="us-east-1"
)
# Configure boto3 bedrock service client
bedrock_client = boto3.client(
service_name="bedrock",
region_name="us-east-1"
)
# Initialize Javelin Client
config = JavelinConfig(
base_url=os.getenv('JAVELIN_BASE_URL'),
javelin_api_key=os.getenv('JAVELIN_API_KEY')
)
client = JavelinClient(config)
# Passing bedrock_client is recommended for optimal error handling
# and request management, though it remains optional.
client.register_bedrock(
bedrock_runtime_client=bedrock_runtime_client,
bedrock_client=bedrock_client,
route_name="bedrock" # Universal route for the Amazon Bedrock models
)
# Example using Claude model via Bedrock Runtime
response = bedrock_runtime_client.invoke_model(
modelId="anthropic.claude-v2:1",
body=json.dumps({
"anthropic_version": "bedrock-2023-05-31",
"max_tokens": 100,
"messages": [
{
"content": "What is machine learning?",
"role": "user"
}
]
}),
contentType="application/json"
)
response_body = json.loads(response["body"].read())
print(f"Invoke Response: {json.dumps(response_body, indent=2)}")
# Example using Langchain
from langchain_community.llms.bedrock import Bedrock as BedrockLLM
llm = BedrockLLM(
client=bedrock_runtime_client,
model_id="anthropic.claude-v2:1",
model_kwargs={
"max_tokens_to_sample": 256,
"temperature": 0.7,
}
)
stream_generator = llm.stream("What is machine learning?")
for chunk in stream_generator:
print(chunk, end='', flush=True)
JavaScript/TypeScript
- OpenAI
- Langchain
- Bedrock
- ...
npm install openai
import OpenAI from "openai";
const openai = new OpenAI({
apiKey: process.env.OPENAI_API_KEY,
baseURL: "https://your-api-domain.com/v1/query/{your_route_name}",
defaultHeaders: {
"X-Javelin-apikey": `${process.env.JAVELIN_API_KEY}`
},
});
async function main() {
const completion = await openai.chat.completions.create({
messages: [{ role: "system", content: "You are a helpful assistant." }],
model: "gpt-3.5-turbo",
});
console.log(completion.choices[0]);
}
main();
npm install @langchain/openai
import { ChatOpenAI } from '@langchain/openai';
const llm = new ChatOpenAI({
openAIApiKey: process.env.OPENAI_API_KEY,
configuration: {
basePath: "https://your-api-domain.com/v1/query/{your_route_name}",
defaultHeaders: {
"X-Javelin-apikey": `${process.env.JAVELIN_API_KEY}`
},
},
});
async function main() {
const response = await llm.invoke("tell me a joke?");
console.log(response);
}
main();
import { BedrockRuntimeClient, InvokeModelCommand, InvokeModelWithResponseStreamCommand } from "@aws-sdk/client-bedrock-runtime";
const customHeaders = {
'X-Javelin-apikey': JAVELIN_API_KEY
};
const client = new BedrockRuntimeClient({
region: AWS_REGION,
// Use the javelin endpoint for bedrock
endpoint: JAVELIN_ENDPOINT,
credentials: {
accessKeyId: AWS_ACCESS_KEY_ID,
secretAccessKey: AWS_SECRET_ACCESS_KEY,
},
});
// Add custom headers via middleware
client.middlewareStack.add(
(next, context) => async (args) => {
args.request.headers = {
...args.request.headers,
...customHeaders
};
return next(args);
},
{
step: "build"
}
);
// Query the model
const payload = {
anthropic_version: "bedrock-2023-05-31",
max_tokens: 1000,
messages: [
{
role: "user",
content: "What is machine learning?",
},
],
};
const command = new InvokeModelWithResponseStreamCommand({
contentType: "application/json",
body: JSON.stringify(payload),
"anthropic.claude-v2:1",
});
const apiResponse = await client.send(command);
for await (const item of apiResponse.body) {
console.log(item);
}
We have worked on the integrations. Please contact: support@getjavelin.io if you would like to use this feature.
Authentication
All requests to Javelin endpoints require authentication using:
- Javelin API Key: Passed in the
X-Javelin-apikey
header - Model Provider API Key: Passed in the
Authorization
header (mostly)
Example:
curl -X POST "https://your-javelin-domain.com/v1/chat/completions" \
-H "Content-Type: application/json" \
-H "Authorization: Bearer YOUR_PROVIDER_API_KEY" \
-H "X-Javelin-apikey: YOUR_JAVELIN_API_KEY" \
-d '{ ... }'
Javelin Headers
When integrating with Javelin, you can customize behavior and routing by using the following custom headers in your requests:
Header | Required | Description |
---|---|---|
X-Javelin-apikey | ✅ Yes | Your Javelin API key. Used to authenticate requests to the Javelin platform. |
X-Javelin-virtualapikey | Optional | A secure placeholder for provider API keys. Instead of exposing real provider credentials, you can pass a virtual key ID; Javelin will resolve it to the real secret stored in your vault. This adds security, supports credential rotation, and simplifies integration. |
X-Javelin-route | ✅ Yes (for unified endpoints) | Specifies the Javelin route name to determine model, provider, fallback policies, and guardrails. |
X-Javelin-model | Optional | Allows overriding the default model at runtime (e.g., gpt-4, claude-3-sonnet) for OpenAI-compatible or Bedrock-like APIs. |
X-Javelin-provider | Optional | Use this to override or specify a provider URL (e.g., https://api.openai.com/v1). Useful in cases where your route supports multiple backends. |
⚠️ Some headers are optional depending on the type of endpoint (OpenAI-compatible, Bedrock, Azure, etc.), but using
X-Javelin-apikey
andX-Javelin-route
is standard for most integrations.
Error Handling
Javelin returns standard HTTP status codes and error messages. Common errors include:
Status Code | Description | Common Causes |
---|---|---|
400 | Bad Request | Invalid request format or parameters |
401 | Unauthorized | Missing or invalid API keys |
404 | Not Found | Endpoint or route doesn't exist |
429 | Too Many Requests | Rate limit exceeded |
500 | Internal Server Error | Server-side issue |
Example error response:
{
"error": {
"code": "401",
"message": "Invalid request: model parameter is required"
}
}
Best Practices
- Use Environment Variables: Store API keys in environment variables, not in code.
- Implement Retry Logic: Add retry mechanisms for transient errors.
- Set Timeouts: Configure appropriate timeouts for your application needs.
- Monitor Usage: Use Javelin's monitoring features to track usage and costs.
- Test Thoroughly: Test your integration with different providers and models.
Troubleshooting
Common Issues
-
Authentication Errors
- Verify both provider and Javelin API keys are correct
- Check that keys are passed in the correct headers
-
Endpoint Not Found
- Confirm the endpoint URL is correct
- Verify the route exists in your Javelin configuration
-
Request Format Errors
- Ensure your JSON payload matches the expected format
- Check for required fields specific to the model you're using
-
Rate Limiting
- Implement exponential backoff for retries
- Consider adjusting your request patterns